Blog
Post-Event Report: Building Beta Data Management Protocols for Soil Carbon GHG Quantification
To assist USDA in initial designs for their “Greenhouse Gas (GHG) Quantification Program,” Purdue Open Ag Technology and Systems Center (OATS), Semios, The Mixing Bowl, and Farm Foundation hosted the Building Beta Data Management Protocols for Soil Carbon GHG Quantification virtual “event storming” which took place on October 24, 2023.
Summary of the Meeting Discussion
Modeling and measuring soil carbon content is a soup of complexity. Even deciding what to measure and how to measure it is difficult enough. Add to that the sometimes difficult to quantify variations in sampling protocols, labs, model needs, the meaning of terms, and the humans involved. And add to that the social and coordination complexities of differing goals across necessary stakeholders such as landowners, government, and industry, interoperability across many organizations for both lab results and records of farming practices, and the sheer spatial scale of estimating soil content across the entire country.
If that wasn’t enough, the models of the relationship between farming practices and changes in soil carbon content are themselves under active research and development which will likely lead to innovations that may constantly move the goalposts for data collection. Much like actual soup, this “complexity soup” must be eaten one spoonful at a time, and more spoons working together will get to the bottom of the bowl faster. Success will require both generalized coordination and cooperation that builds a solid community of contributors as well as specialized early pipelines that get the first data flowing quickly as a basis to start iterating improvements.
What follows in this document is:
- Background on the Event Storm Activity
- Four Segment Timeline Overviews
- Summary of the Event Storm
- Identified Focus Areas & Ideas
- A Suggested Path Forward
- Appendix: References, Keyword Clusters & Tags
On Tuesday October 24, 2023, a diverse group of government, industry, and academic pioneers gathered for an “event storming” session to gain a shared understanding of the issues involved and solutions for “beta data management” systems involved with measuring soil carbon content and modeling the relationship between farming practices and change in soil carbon content, with a special focus on the United States Department of Agriculture (USDA) initiative to perform country-wide soil sampling and data collection which collects existing data, creates new data, and connects data with carbon models and researchers. Over the course of just a few short hours, the group had collectively produced an online whiteboard with over 2,000 pieces of information toward this goal.
The goal of this event was to form a community-led understanding of data management surrounding the measurement of soil carbon content and to identify common pain points to be addressed in follow-up events and initiatives to help solve them. Participants jointly identified actions, actors, and data exchanges that occur, or need to occur — through a method known as “event storming.” The resulting Miro board with the virtual sticky-note-based results can be found here.
Event Storming Background
Traditional “event storming” tries to understand the big picture of a process by focusing on the “events” that take place and their approximate order in time. In a pre-pandemic world, this meant writing past-tense verbs (“events”) on orange sticky notes and sticking them on a wall as a big, in-person group of domain experts. In an attempt to approximate the rich conversational environment of in-person sessions, the supply chain was segmented into four separate but overlapping “sector timelines”: groups of sticky notes designed around optimizing a particular group of activities or goals. The larger group of event stormers was broken into four groups, each rotating through those segments while placing stickies and sharing their experiences and insights with the group. The four sector timelines were: Field Data Collection, Soil Lab, Feeding the Models, and Metadata Management.
In addition to events (represented with orange stickies), purple stickies represent “hot spots”: disagreements, ambiguities, etc. White stickies indicate “terms” that arise which comprise part of the domain language.
Finally, once the basic event flow has been established, blue stickies are added to represent decisions, questions, or “triggers” that are relevant to the people involved with the events in the timeline. The people themselves are shown on yellow stickies, and any data or information needed to make those decisions, answer those questions, or pull those triggers was added on green sticky notes.
The resulting “big picture” timelines give insight into what happens, who is making decisions, what questions need to be answered, and what data is needed at which points in time.
Segment Timeline Overviews
The virtual small groups succeeded in triggering some robust conversations, and the group as a whole produced rich event maps. We had a large and diverse group of participants, including farmers, agricultural service providers, ag tech companies, USDA personnel, academics, and researchers. The broad expertise and differing windows through which participants viewed various parts of the problem resulted in a lot of knowledge sharing and great progress was made toward the goals of developing a shared understanding of the concepts and issues facing beta data management. Of particular note was the unexpected poetic realization that rutabagas, despite encompassing a negligible amount of land use, have a particularly lyrical quality of “beta rutabaga data.”
FIELD DATA COLLECTION
Moderator: Prof. Ankita Raturi, Agricultural Informatics Lab & Open Ag Tech and Systems Center at Purdue University
When discussing field data collection in the context of soils in agricultural landscapes, the first image that comes to mind is a clod of rich, dark, fresh earth. We think of a laptop computer perched precariously on the edge of a truck, a trail of tests and emails, and the eventual chaos of wrangling a suite of datasheets from clipboards and electronic documents to start the journey. However, the lifecycle of a soil sample begins months, sometimes years, before it is collected, with discussions among farmers and other land stewards, researchers and technical assistance providers, extension agents and crop advisors as they establish a shared project goal and subsequently coordinate and collaborate an intricate sequence of field data collection events. This breakout session mapped three event clusters where these soil data stakeholders collaborate to determine: (1) how a soil field data collection plan is developed, (2) how sampling logistics are coordinated and executed, and (3) how the soils data are verified, validated, and ultimately prepared for downstream analysis and use.
Establishing a Project Goal
Some land stewards sample soils on their fields for their own understanding, using ad-hoc or on-demand data collection methods that likely have simpler logistics as they work independently to quickly ascertain some basic conditions of their field: pH, soil moisture, or otherwise, to inform an in-situ or in-season decision. However, land stewards increasingly participate in larger projects, where soils data are collected across landscapes as part of a goal-driven sampling project: to quantify soil carbon and GHG, to understand the movement of nutrients, and so on. In this scenario, the field data collection logistics are more complex. Individual land stewards must collect data using near-identical protocols, measure the same soil attributes, and even use the same labs for testing (as discussed in a separate session during this event). This level of coordination and collaboration inherently demands a more formalized methodology that is typically created through a rigorous planning process among soil data stakeholders.
In this session, participants converged on mapping this latter form of goal-driven and coordinated soil data collection, describing a semi-formal process where groups of data collectors are oriented toward common scientific questions. Individual data collectors, such as the land stewards themselves, will often have complementary site-specific goals related to soil health and land management. In this sense, the purpose of soil data collection is both to enhance scientific understanding on soil carbon and GHGs, as well as to improve management practices to support sustainability outcomes.
Once a group of stakeholders are identified (e.g., land stewards, technical assistance providers, government regulators, scientists), a project manager will meet with the group to be able to establish specific, scientifically-feasible, and practical project goals. Participants noted the importance of thinking on protocol as an artifact with many parts: sampling methods, stratification strategies, data structures and vocabularies. These different components are informed by, of importance to, have impact on, and subject to constraints by, different stakeholders, which means that there is need to ensure clear communication, transparency, clarity, and consensus among the project stakeholders.
Background Data Preparation
Next, the project stakeholders need to determine who they need to consult to inform the protocol, as well as identify which data are needed to develop the protocol. In a listing exercise, participants named a non-trivial initial set of data they think are necessary for protocol design: field boundaries and field-level data; tract, PLU ecological site ID and other land identifiers; soil conditions including composition, texture and suborder; historical cropping history including crop rotations, planting and harvest dates; management practices including input application and tillage history (with amount, data, and type for each); existing and planned conservation practices; land use, vegetation, natural resources, drainage, hydrological groups and other environmental conditions; and probably more.
This significant baseline data creates a slew of complications. First, participants noted that there is a desire to identify what a “minimal” dataset constitutes and to come to a consensus across different soil data collection efforts on the necessity and utility of different data types. Digital field-level data, with semantic integrity is also hard to obtain. Each of the different types of data is collected for multiple, sometimes conflicting purposes: for crop management, for regulatory reporting, for ad-hoc understanding, and sometimes, the data simply does not exist. Data quality, format, structure, and reusability are also subject to the norms and limitations imposed by the data collection tools used. This varying level of data availability, granularity, and standardization means that the seemingly innocuous task of collecting background data to inform a soil data collection plan can devolve into a behemoth data science project in itself.
Create the Standard Operating Procedure (SOP)
Once the required background data are collected and prepared, they are used by project stakeholders to create an SOP or protocol. Three questions drive this event. What data needs to be collected and through which sampling methods? What is the stratification goal and strategy? Who will collect the data? Participants noted the need to strive for simplicity in the sampling and data collection process to improve data quality, consistency, and practicality.
Participants dug into the process for identifying a stratification methodology, as it is a critical component of a protocol design. At a minimum, a set of field boundaries must be shared for stratification. These boundaries, in conjunction with any other field-level data available, are used to create a soil sampling plan. The field maps may be compared with the background data to establish areas of variability and priority. The stakeholders must make many highly granular decisions, for instance: which soil characteristics are of key interest to measure; sampling hardware and how the act of sampling needs to occur (e.g., sampling depth, density, number); which labs to use for subsequent soil testing; and how to accommodate site-specific constraints (e.g., physiographic limitations). Once these decisions are agreed upon, stakeholders prepare appropriate data layers, sometimes conducting a mini-quality assurance process to determine data suitability and validity. Choice of stratification algorithm then depends on further decision points such as composite vs single core sampling requirements; project-level vs field-level stratification, and the feasibility of the proposed sampling effort.
Once a stratification and sample plan is created, several forms of stakeholder consultations must occur. Land stewards and field techs are consulted to understand site-specific constraints and, in an ideal situation, the SOP is customized for different sites through a common approach. The SOP documents are ideally shared with the scientific community for review if there are radically novel approaches utilized that have yet to be scientifically validated, as is often the case given the emerging soil science and related research. Participants noted that project stakeholders would ideally also consult with registries, particularly if the land stewards involved are interested in carbon credit and other ecosystem service marketplace programs. Similarly, consultation with agencies like NRCS involved in conversation planning to ease the downstream burden of cost-share program or regulatory reporting. Participants noted that while we often treat the SOP as though it is set in stone, it is typically modified once the sampling venture commences as things change. As SOP review with different stakeholders comes to a close, the level of granularity in an SOP may be increased further to provide step-by-step guidance for in-field staff and technicians who may actually conduct the soil sampling. The project stakeholders both create training materials and ideally conduct in-field training with soil samplers on how to enhance the SOP.
Given the gargantuan effort involved in protocol design, many questions remain. Does planning always translate to action? What is the minimal effort to create a scientifically valid field data collection SOP to understand soil health, quantify soil carbon and GHGs, and ultimately support improved land management actions.
Sampling Logistics
If a detailed, coherent and practical field data collection protocol is designed, then the actual in-season logistics of coordinating sampling dates with land stewards, and the actual act of sampling itself, are more straightforward. Sampling is scheduled with the land steward, or if their own team is responsible for the sampling, they coordinate amongst themselves. A trained field technician arrives at the site, plans their route among all the sample locations, and then simply samples according to plan at the predefined locations. Ideally, there is guidance in the SOP on how to adjust if there is something unexpected at the location itself (e.g., wet, too steep, there’s a tree!). The SOP should also contain guidance on how to select subsamples within the field.
The biggest pain point during the field data collection itself is ensuring that samplers collect the necessary metadata to ensure that the samples are not just meaningless, uncontextualized lumps of soil. Field-level data is ideally collected first, including land management with this-season conditions (e.g., harvested/current/intended crop). Samples, including subsamples, are ideally located to some level of positional accuracy and include, for instance, an interpretable sample ID, GPS data, collection date, sampling depth, soil conditions, type of sample (e.g., bulk or carbon), implement used (e.g. shovel or probe). The sample must also be bagged, tagged, and geolocated according to the lab where it will be sent. Participants noted the need for some level of quality control to be conducted on the sample data before leaving the field, including annotations of possible errors or changes.
Once the samples are collected, those that will be subject to lab testing require work orders to be verified and shipped with the samples to the lab. At this point, there is a suite of soil data events and challenges that occur on the lab-side, as discussed in the “Soil Lab” breakout group.
Data Verification, Validation, and Handoff
A common aphorism in statistics is that “all models are wrong, but some are useful. Participants noted, that similarly, all data are wrong, and the challenge lies in determining how to quantify how “wrong” the data is. That is, when we collect any data, there is some degree of error or uncertainty. Participants noted the importance of verification of soil data samples: for instance, bulk densities are very error prone in practice depending on how the sample was collected. While not all uncertainty can be accounted for, it is important for documentation and metadata to reflect how and where these issues may crop up. Data are also subject to bias (e.g., in where it was sampled); thus, it is important to ensure that there are some mechanisms to document assumptions and constraints in Quality Assurance and Quality Control (QA/QC) plans. There is also a need for methods to reduce errors as data and samples are passed from sampler to shipper to lab tests and back. Solutions may include simply tagging samples themselves with supplementary key identifiers in case of mixups. Sample documentation could also be linked to the lab results and site data.
Where data verification deals with accuracy and quantifying uncertainty, data validation involves ensuring that the data reflects the initial protocol adequately. A simple and common data validation example involves checking to see that the number of samples actually collected match the prescribed number of samples, and if not, why. However, participants noted the need for validation of the process itself! This could be in the form of community review of processes or some form of external review from analogous data stakeholders.
Once data is verified and validated, the issue of how to communicate that a sample is “certified” arises. Particularly as the data assembly process begins and different types of data with varying levels of accuracy, uncertainty, and quality are interconnected, there is a need to ensure data traceability back to the point of origin. As a field data collection wraps up, stakeholders may consolidate data into a master datasheet, upload it to a shared database, or simply file it away in a physical or digital folder. However, the reality of field data assembly also includes a mess of data spread across email inboxes, various record management systems, individual phones and computers, and many other formal and informal locations. Participants noted that many of the challenges in data assembly include the need for improved interoperability, whether through the adoption of compatible semantic standards or tools for data conversions. As discussions regarding data sharing begin among data stakeholders, issues around privacy, trust, governance, consent management, and concerns regarding FAIR data must be considered. The downstream complexities involved in using soils data and handling metadata were further discussed in the “Feeding the Models” and “Metadata Management” groups, respectively.
SOIL LAB
Moderator: Aaron Ault, Open Ag Tech and Systems Center at Purdue University
A natural assumption in the soil lab segment was that it starts after the samples have been collected in the field. However, the “soil lab chosen” event made it clear that several parts of the timeline happen prior to digging in the dirt. The timeline sorted itself into roughly three categories: prior to the soil sample arriving at the lab, between arrival and testing, and reporting of results.
Prior to Lab
Some information from the “prior to lab” stage needs to “leapfrog” the lab: i.e., the lab doesn’t care about them and doesn’t want the privacy implications of holding them, but the eventual models need such information. Examples include the sampling locations (ideally GPS), environmental conditions during sampling, sampling protocols (including how cores were taken or combined), farmer practices (cover cropping, etc.), and potentially farmer sentiments on the location via questionnaire. Clearly, samples need identifiers that pass to the lab and then through the lab to line up results with this leapfrog data later. This sort of data transfer generally happens already, either a priori in anticipation of a box of samples arriving at the lab or directly in the box itself. This has a serious design implication in that if labs are chosen later to report results directly to USDA, the lab may in fact need to collect and relay information that they would rather not mess with. It also means that the creator of the initial ID for a sample should be well-defined in the overall process.
Several participants voiced concerns about the consistency of lab results in practice. Some of this may be due to inconsistencies in sampling methodology, including efforts prior to collection. Adoption of scientifically grounded standard operating procedures, such as those developed by the Soil Health Institute, is recommended to improve consistency and reliability of collected data. Labs generally adhere to national Quality Assurance/Quality Control (QAQC) protocols administered via several potential agencies. However, this sort of QAQC happens prior to sampling and so is more generalized than the quality of results for a single box of samples. It therefore does not seem to guarantee the absence of testing anomalies on any given day. To combat this, the preferred method voiced among participants was to include duplicate soil samples (i.e. split the same cores into multiple bags) in each set of samples. This has the disadvantage of added cost, but the advantage of minimal coordination: only the person sending the samples needs to participate in the protocol in order to know what level of trust the resulting data should have.
Variations in lab logistics can cause some headaches for a national-scale program: some labs have specific Standard Operating Procedures for samples, such as the minimum amount of soil and type of bag used, which can provide practical barriers to nationwide consistent protocols. In addition, labs themselves may support different test assays with different methods. The MODUS standard hosted by Ag Gateway provides an excellent suite of test codes for clear, specific test assays and this data should certainly travel along with the lab results themselves. This only identifies the test performed; however, an additional layer of requirement likely needs to specify which type of tests are allowed in order to participate. Clearly, a lab certification component will be necessary for a national scale sampling program.
At the Lab
Once the box of samples arrives at the lab, the lab will generally scan QR codes on bags or otherwise record the identifiers for samples. Each sample’s requested assays can be included in the box or transmitted ahead of time and associated with the sample ID. It is unclear what level of data about samples in transit will be relevant, but some questions do exist, such as time spent in transit, temperature in transit, time in storage before testing at the lab, etc.
The lab may homogenize a sample during testing, and the method used and resulting soil properties (texture, particle size, moisture content, etc.) may be important to record.
Some kinds of assays are more advanced and uncommon than others. A baseline suite of test assays should be defined for generally available, inexpensive tests, and value-add suites of tests can also be performed in certain cases as warranted. The phrase “super sites” was used to describe soil sampling sites which may consistently order more advanced tests. From a modeler’s perspective, this is important because it means there will be a large dataset with simpler data, and a smaller dataset with more detailed and advanced data. It is also possible that new methods for testing may become relevant throughout the program’s life cycle, so a framework for adapting to changing test schemes should be developed, ideally with an eye to understanding the comparability of old procedures to new ones in order to maximize the size of usable datasets.
Reporting
In general, labs report results to the person who sent the sample (and paid for it). Reporting in the MODUS standard should be required for any national scale program as it will ensure interoperability among data and a clear means of identification of lab test procedures. Existing reporting tends to be missing critical data about the actual tests that were performed to get the results, and they are generally haphazardly organized in non-standard CSV’s or spreadsheets. Work is ongoing on an open source tool and code libraries (https://oats-center.github.io/modus) to make it easier to transition between spreadsheet and the MODUS standard. Should a MODUS-based interoperability come to fruition in the soil health industry, it will finally enable the community to build tools and services that can deliver value to large swaths of stakeholders, including farmers, landowners, researchers, etc.
There is as yet an unclear path from lab to model researcher. Some participants may be interested only in feeding their data into an existing model for a carbon certification, while others may be more interested in enabling research into the models themselves. Modelers would prefer a reasonably centralized repository of hosted data with clear privacy and use restrictions. This introduces a single point of failure for any participants involved in the sample collection side, and could be a very onerous privacy burden on the host of the data. Centralization is also often inherently less secure since it allows malicious actors to focus on a single platform. It may be that a hybrid approach leveraging interoperability among a network of data sites could provide a reasonable best of both worlds.
To the extent that the program wants to assemble data beyond prescriptive, paid-for direct sampling, some consideration should be paid to how to encourage participation. The set of potential soil testing labs is a much smaller target than the full set of all landowners and farmers, and therefore may provide a better avenue to adoption. A lab could be set up to provide a copy of results directly into a data collection platform as an add-on feature for a sample, thereby enabling them to also provide awareness to their customers of the program. However, this will still need to solve the problem of the “leapfrog” data which the lab does not historically collect.
If the person who sent the sample to the lab intends for the sample to go directly into a model such as COMET, there may also be an opportunity to streamline that process as well.
FEEDING THE MODEL
Moderator: Rob Trice, founding partner of The Mixing Bowl & Better Food Ventures
The primary purpose of the USDA model is to improve our understanding of management practice efficacy to sequester GHG by providing estimates as accurately as possible. The two most important things about the model are that 1) it possesses the ability to ingest comprehensible information through data structures and ontologies it can understand, and 2) it possesses the ability to be updated so that over time it can be even more accurate by collecting and connecting with other, new data.
We know that, through research and data analysis, we will learn more about things like farm-level practice implementation. I.e., what will the impact of management practices–like cover crop application– be on soil carbon sequestration? We also know that additional soil analysis data will be added to the model in the future.
We also know that USDA will start building its model based on COMET and DayCent. COMET is a greenhouse gas accounting tool that is used to estimate greenhouse gas emissions and carbon sequestration from agricultural production. DayCent is a biogeochemical process model that is used to simulate soil carbon and nitrogen dynamics, as well as greenhouse gas emissions. COMET uses DayCent as its underlying model to simulate entity-scale greenhouse gas emissions. This means that COMET relies on DayCent to provide estimates of greenhouse gas emissions from various agricultural activities, such as crop production, livestock production, and manure management. COMET also provides users with the ability to input their own farm-specific data, such as crop types, management practices, and soil conditions. This allows COMET to generate more accurate estimates of greenhouse gas emissions for individual farms.
The USDA model will leverage COMET and DayCent and will be able to ingest new data from the field, from labs, from scientific researchers, and from others’ models and databases.
Harmonizing Data Collection
To be effective, both the model, and those contributing data to it, need to have a common set of terms and data fields to feed the model. For instance, looking at the example of cover crops, we need common terms to describe what was planted (legume or non-legume?), when, where, and, additionally, when the cover crop was terminated.
Exact data structures and semantics need to be communicated to those capturing data to be used in the model.
Defining “Required” Versus “Desired” Data
There is a natural tension that exists between making the model functional through ease of data collection by focusing on only a minimal set of “required” data versus also capturing potentially important (“desired”) information for the future. The qualified data collectors who are gathering field or lab data need to know clearly what to capture.
As an example, in addition to soil organic matter or soil organic carbon levels, perhaps we may want to collect information for future modeling related to soil mineral, microbial, DNA, enzyme levels enabled by technologies like portable FT-IR spectroscopy.
Meta Data Management for the Model
In addition to data structures and semantics, we recognized that there is important data that must be collected about the data inputted into the model. For instance, we need to define scales for data capture (meters or inches?), location, time, (tool? method?) of data captured.
Regarding model input, we need to identify a QA/QC process and some methodology needs to be determined to handle “missing data” from imputation mechanisms.
Regarding the output from the model, we need to make sure users know what version of the model was used to determine an output.
While there is another USDA working group– the Model & Tools Group– that will be responsible for measuring the efficacy of the model, determining measurement methods and metrics to assess the performance of the model, we recognize that we need a way for “the model to feed the model.” The model is intended to be dynamic (not static) and improvements to it need to be identified and rolled into the model somehow.
A separate Miro “room” looked at meta data for the overall modeling initiative and we want to make sure that group considers security of the ML models and identification of adversarial AI that could occur through different model harmonization.
Inter-Model Harmonization
We are aware of other international and proprietary efforts to quantify agriculture GHG and we need a way to harmonize or interoperate with those other models to the degree possible. AgMip, an organization established in 2010 with the sole purpose of making agriculture models interoperable and intercomparable, might be someone to partner with in this regard.
The figure below very accurately represents many of the data input and management challenges we identified.
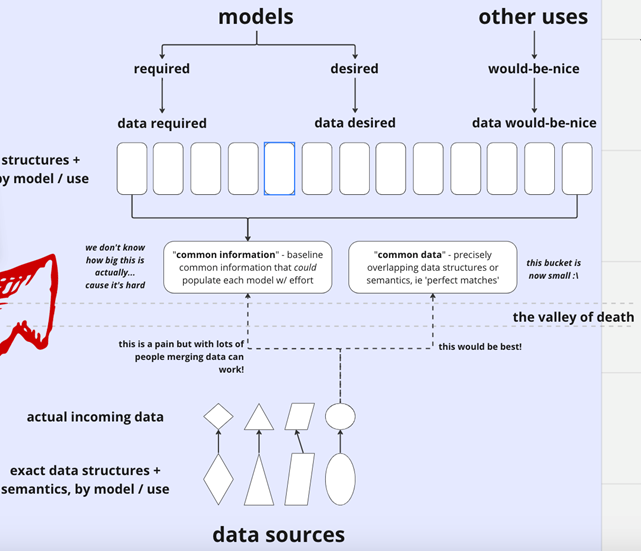
Building a Community of Users
A last important point not to be overlooked is that USDA needs to build a community of model users. Three kind of users need to be accounted for:
Data Collectors & Inputters need to be communicated with so they clearly understand what data to collect and how to properly input data. This would not only include qualified data collectors but soil lab technicians so they understand what and how to analyze data.
Other Modelers need to be communicated with so they can help to harmonize and interoperate models so we get the benefit of more data.
End Users who will use the output from the model will really need to be identified and included in the development of the model. We identified the following end users:
- Other modelers & scientific researchers who will want to leverage the model’s data for their own modeling.
- Certifiers & reporters will want to use the model for purposes ranging from carbon registries to the EPA reporting on US carbon levels to the UN.
- Technical advisors for farms and ranches (like NRCS) who are looking to promote optimal climate-smart agriculture practice implementation that is crop and locale-specific based on the latest science and models.
METADATA MANAGEMENT
Moderator: Drew Zabrocki, co-founder of Centricity by Semios, Advisor, OATS Center at Purdue University and International Fresh Produce Association.
Conversations on Metadata Management underscored the importance of collaboration, data management, and technology in inspiring model creation, insights, and policy to improve soil health practices and promote sustainable agriculture.
The following summary points were raised in our discussions:
- There is a need for collaboration between different organizations and stakeholders, especially industry. There are many systems and standards—working together will assure the best outcomes.
- The need for transparent and extensible frameworks to make data more accessible and comparable.
- There will be changes. We need to incorporate standards and protocols for managing change.
- The use of open-source software and technology can help in data interoperability and standardization.
- The involvement of public and private actors in advancing a solution to meet the needs of all stakeholders.
- The significance of community-driven science and participatory research.
- The potential of soil data measurement, farm management information systems, and mapping technologies to unlock the full potential of the supporting insights tools.
- The importance of data sovereignty and transparency for all stakeholders.
Our discussions were focused in the following overarching areas:
Data Sovereignty
Effective data governance and security require careful consideration of several key factors. These include establishing clear authority levels, documenting data privacy and consent management, defining data rights and obligations for stakeholders, ensuring transparent processes for data sharing, determining ownership, promoting interoperability, automating reporting, and implementing certification frameworks. By implementing these measures, businesses can foster accountability, protect privacy, and inspire trust in their data management practices.
During the discussion, we also delved into important topics such as interoperability, data sovereignty, automated attestation, and the utilization of open-source tools such as the AGAPE certification framework. Additionally, there was a focus on permitting labs to share soil test results with NRCS. We explored some of the challenges and potential solutions in these areas (see References), emphasizing the importance of maintaining control, trust, and collaboration for enhanced management and innovation across various domains.
Correlation & Connecting Systems
Data, with linked documentation and semantic resources, adds valuable context and insights. It can be connected to on-farm data systems and tools, enabling aggregation of metadata at various levels. Engaging with diverse systems assists in research, auditability, and supports predictive and analytical modeling. In this pursuit, prioritizing published and well-documented APIs is crucial. The versatility of data extends to educational programs, rendering it a valuable resource for teaching and learning. In summary, data presents endless opportunities for developing new models, exploration, and innovation.
Creating Value through Sharing & Insights
The agricultural sector holds immense potential in utilizing the available data for various applications like analysis, modeling, and education. Ensuring statistical relevance and correlation through data analysis becomes crucial, which may involve making subsets or derivatives of data accessible to the public. Leveraging existing farm management information systems like Agworld or FarmOS can significantly enhance data quality and reliability. These tools, integrated with accurate and diverse data sources, are invaluable for conducting research and generating obfuscated data for multifaceted purposes.
Throughout these discussions, the importance of implementing continuous improvement frameworks and fostering an interactive process that incorporates stakeholder participation became evident. These conversations underscored the need to embrace opportunities for learning and growth in order to continually evolve and advance.
Event Storm Summary
From soil data measurement and modeling for near-term regulatory needs to AI and machine learning that may unlock new insights, the potential for value creation is vast. Transparent standards, community-driven solution design, and built-in data sovereignty are crucial for all stakeholders.
This comprehensive discussion delved into various key areas crucial for effective data management and collaboration. It placed particular emphasis on standardized units of measurement, capturing and addressing uncertainties, and implementing strategies for continuous improvement. The importance of relating environmental data, establishing robust data governance practices, and seamlessly connecting systems was also brought to the forefront. Additionally, the conversation underscored the potential for unlocking value through information sharing and insights, while highlighting the need for ongoing improvement and active stakeholder engagement. Ultimately, this discussion served as a reminder of the paramount importance of data control, trust, and collaboration in driving effective management and fostering innovation across various domains.
Identified Focus Areas and Ideas
The concluding session of the event used arrows ↩ to denote areas of the board where solutions should be focused, and red stickies to suggest ideas, projects, or paths forward in those areas.
IDEAS FOR FIELD DATA COLLECTION
Participants offered a set of proposed recommendations and existing methods to mitigate the potential of a data preparation boondoggle:
1. Need for improved field data collection systems: The company OurSci takes the approach of creating common “question set” libraries that use community-identified standard vocabularies design to help “pre-align” data inputs with their downstream intended use, as exemplified in their field data collection tools SurveyStack and SoilStack. [axilab user research]
2. Need for improved interoperability among data collection systems: There is a need to adopt common agricultural vocabularies, ontologies, and data standards. Though there will always be stylistic differences among systems, participants note the need for community consensus on how we should be structuring farm data sharing through ongoing efforts to resolve this issue. AgGateway, a consortium of agricultural and technology industry partners, has been developing the ADAPT Framework that consists of an Agricultural Application Data Model, and API, and a suite of data conversion plugins, all designed to meet their set of proposed industry standards to “simplify [data] communication between growers, their machines, and their partners.” The Purdue OATS Center takes yet another approach through [OADA + AGAPEcert].
3. Need for improved participatory data and protocol stewardship process: includes the collection of background data to determine baseline constraints and land status; Need to include more stakeholders in the protocol design process. In a collaborative effort among NRCS, Purdue Agricultural Informatics Lab, OurSci, FarmOS and OpenTEAM needs assessment.
4. Need for open soil data collection protocols: Through this entire process, participants note for the SOP to be a living but versioned document.
IDEAS FOR SOIL LAB
A few focus areas arose from the chaos near the end of the day. The quality assurance process was highlighted as a focus area, with ideas for tackling that as certifications which can be passed along from a lab, and passed along from a set of samples with quality control duplicates which could provide a level of trust with the lab results as they move on through the models.
The largest focus area was around institutionalizing the use of the MODUS standard for soil sampling lab results. An idea for tackling this was to make tools that make it easier for people to use MODUS than not. Building the MODUS community, participating in the Ag Gateway standards committee, adding MODUS requirements for program participation, and building open source tools and libraries are all in the mix. Though it was in a different area of the board, there was a suggestion to build a database of supported bulk density methods and soil carbon methods used by specific labs which is an ongoing critical effort around the MODUS standard enabling the existing open source tooling to work with many different labs’ reporting.
Finally, in order to kick start the process, the suggestion was made at the “reporting to a centralized data repository” level to create an open source, redeployable implementation of a potential centralized platform. This way, the API’s and schemas can be initialized and iterated across parallel proof-of-concept pipelines, while maximizing the likelihood that an eventual centralized platform (or network of platforms) will easily interoperate with smaller-scale early developments and industry platforms.
IDEAS FOR FEEDING THE MODELS
We identified four summary actions to be taken:
- Define what data needs to be collected by data collectors or inputters and define the common data structures, ontologies, and associated metadata for model data. This includes defining a minimum data set necessary to start the model, additional data you might want to collect now for analysis, and also data you might want to collect in the future.
- Develop a QA/QC process for data imputed into the model and develop policies for handling “missing data.”
- Develop a process for harmonization or interoperability between this model and other models.
- Develop “user communities” to make sure their needs are captured in the development of the model. Three kinds of users were identified:
1) Data collectors & inputters to feed the model,
2) Other researchers & modelers who may want to collaborate to refine the model, and
3) End users who will utilize the output from the model.
IDEAS FOR METADATA MANAGEMENT
We identified the following actions to be taken:
- Establish a comprehensive data dictionary detailing variables and units. Assistance for this project is available through the IRA-GHG initiative. For further details, please visit AgGateway’s website at www.aggateway.org.
- Create a hierarchical data measurement protocol that’s been expertly validated. The aim is to seek a flexible solution that’s neither overly prescriptive nor restrictive.
- Develop comprehensive guidance on metadata governance, security, and privacy by engaging all relevant stakeholders, including IT, legal, and technical experts. It is crucial to fully comprehend the intricacies of data privacy, consent management, and the rights and responsibilities of various parties involved.
- Explore and outline data sharing protocols, data ownership, and the challenge of maintaining interoperability while upholding data sovereignty. Seek out industry best practices and leverage open-source tools for automated reporting, utilizing the OODA LOOP framework. For validation of claims without compromising sensitive information, consider the implementation of the AGAPECert automated certification framework.
- Identify and resolve the need to manage uncertainty through the capture of temporal-geolocation information. Additionally, there is a requirement to record and report the measurement of uncertainty in the data.
- Determine publicly available data related to soil, which can be connected with other data available from stakeholders likely available in farm management systems (FMS). Develop methods for how the soil data can be reliably associated with on-farm metadata such as fields and boundaries. Map use cases for how the data is aggregated at different levels, including farm, regional, and national, to assist in frameworks and guidance documents.
A Suggested Path Forward
Despite the number of branches we found on this complex tree, we certainly identified some low-hanging fruit ripe for the picking. The issues at hand are broad and solutions will inevitably affect a diverse group of differing interests (government, researchers, modelers, farmers, ag service professionals, carbon markets, etc.). The picture is therefore like a wide panorama, and while a panorama can be beautiful, its beauty can be distracting and even paralyzing: the bigger the picture the longer you stare at it. Panoramas are best built as a series of interconnecting pieces: each individual piece seems much more tractable than the whole, so there is much less time needed for admiring the problem’s complexity. If each piece is built in isolation, the picture will never come together in the end, but if one looks only to “the big picture,” they can never get any piece of it actually done.
The key to success here is therefore both parallelism (building pieces of the picture) and end-to-end design (build a crude “total picture” to inform the design of the pieces). The connection points between the pieces represent data interfaces between players and will become the lingua franca that glues it all together. One cannot successfully design such things without the necessary feedback loop of hypothesis and verification: i.e., we think this is mostly the right model, now let’s try it as a proof of concept and see where we’re wrong so we can modify the model and try again.
To that end, the lowest hanging fruit that came out of this event was the idea of focusing on a full end-to-end, narrow use case, and building a toolset in the community that enables coordinated development across more use cases in parallel.
The narrow use case proposed was dubbed “Corn-to-Comet”: collect some actual soil samples and specific practice data (cover crops?) on a few actual fields that produce corn, get that data to the COMET model, get the COMET outputs back to the stakeholders, and end with an overall dataset in a form that could be available for other models and model researchers, all with appropriate consideration of reasonable privacy and use rights. The actual crop/fields chosen for this pipeline should reflect whoever is willing to participate, so the crop may or may not be “corn” in the end.
Backstopping this effort would be the open source development of a deployable “USDA platform” concept. The final outcome of the overall picture will involve reporting soil samples and practice data to a USDA-maintained system which can make such data available to model researchers. With this “redeployable data platform,” individual developers or projects can stand up their own instance of what an eventual USDA platform would look like using consistent schemas and build their own pieces of the puzzle to interface with their instance of the platform. Versions of the schemas and platform code will then serve as an incremental integration test between the various pieces, and its open source nature provides the feedback channel necessary for the big picture to come together. The “Corn-to-Comet” pipeline would use this proof-of-concept tool, and as additional early pipelines are begun from other crops to other models they have some help and examples to get started.
Finally, to keep the valuable insights of the broad tent of community that participated in this event, a future event should be planned which gives participants a chance to talk through design issues with a parallel “hackathon” or “collabathon” among developers to build out real parts of the corn-to-comet pipeline as well as make some proof-of-concept new tools for other pipelines.
Appendix: References, Keyword Clusters & Tags
We made an effort to capture references, keyword clusters and tags related to the event storm activity. Many participants highlighted collaborations already underway that should be evaluated.
AGAPECert Certification Framework: The AGAPECert framework is an automated certification system that aims to validate claims, exchange derivatives, and link related data across domains without revealing private data. This could be a useful solution for ensuring trust and transparency in various domains where certification or complex security policies are required.
AgGateway: AgGateway is a non-profit industry consortium focused on agricultural data interoperability and standards. They manage the MODUS lab test data standard, which is widely used for reporting soil test results.
Agworld: The Agworld ecosystem allows you to collect data at every level of the operation and share this data with everyone that matters. Agworld operates on over one hundred million acres across a broad range of commodities and environments. APIs and integrations with leading technology providers enable all stakeholders to work together on the same set of (field tested) data.
Collaboration and Innovation: It’s important to provide an opportunity for public and private actors to collaborate and find innovative solutions that promote better science, new innovations, and sustainable agricultural practices.
Community Engagement: The team emphasized the importance of community-driven design, research, and engagement.
Data Infrastructure and Management: The IRA-GHG Quantification Program aims to harmonize data for scientific standards and interoperability. Coordinating feedback, developing technical specifications, and creating infrastructure for data management are key aspects of this program.
Data Interoperability & Sovereignty: Standardizing protocols and developing open-source software can help improve data sharing and integration across different stakeholders. The Trellis Framework from Purdue University’s OATS (Open Ag Technology & Systems) Center was referred to as a resource for practical MODUS tools and the OADA and AGAPE toolsets for sovereign interoperability at scale.
Farm Foundation: Farm Foundation is a non-partisan, non-profit dedicated to accelerating people and ideas in agriculture. Their mission is to build trust and understanding at the intersections of agriculture and society by creating multi-stakeholder collaborations. Their strategic priority areas are digital agriculture, market development and access, sustainability, and farmer health.
OpenTEAM: OpenTEAM is an open technology ecosystem for agricultural management that aims to facilitate data interoperability and community-driven science. It brings together stakeholders from various sectors to collaborate on improving data systems in agriculture.
SoilBeat and EarthOptics: SoilBeat and EarthOptics are companies that leverage AI, machine learning, and real-time data mapping to provide insights into soil health. Their technologies help agronomists and farmers make informed decisions about nutrient management and regenerative practices.
Soil Data Management: The National Soil Survey Center and USDA-NRCS play a crucial role in collecting, processing, and delivering authoritative soil data. Their work supports conservation planning and land management efforts.
Soil Health Tech Stack: The “Soil Health Tech Stack” is a term coined by Seana Day in an article that outlines the challenges she sees based on, among other things, her work co-authoring the USFRA Transformative Investment report about how technology and finance could scale climate smart, soil-centric agriculture practices as well as on information gathered during the Farm Foundation Regenerative Ranching Data Round Up. The “Fixing the Soil Health Tech Stack” activities will build upon those efforts and others. As such, the event will leverage pasture/rangeland data but with the goal of extending solutions to all soil-based agriculture production ecosystems.
Tags
#data #comparability #defining
- It is important to agree on data standards and make it someone’s primary job to ensure data comparability
- Developers play a crucial role in defining data models and schemas
- Define data rights and obligations for different stakeholders
- Determine how data will be shared and who owns the data
#design #database #structure
- Design a flexible database that is not too prescriptive/restrictive
- Design the database structure effectively
#measurements #define #accuracy
- Consider using ISO19156 framework for observations and measurements
- Explore ways to upscale measurements
- Define units of measurement for reporting and storage
- Define measurements of uncertainty and accuracy for field measurements
#protocols #data #expert
- Data lineage on lab results and field data should be published as part of versioned protocols
- Publish metadata standards as protocols
- Develop a data measurement protocol hierarchy vetted by experts
- End-users of data may require specific protocols for sampling
#documented #data
- QA/QC standards should be documented to ensure data quality
- Documentation should be linked to other data products for easy reference
- Data collection purpose should be clearly documented
- Data sample design should be documented to ensure representativeness
- Data schemas should support inclusion of existing public datasets
- Provide detailed documents to help users navigate the data
#changes #year #data
- Implement change control for data schema/model changes
- Handle fiscal year/periodic changes in data
- Plan for iterative improvement process in February
- Develop a data variable dictionary for easy reference
#standards
- Consider ISO standards in database construction
- Leverage the good aspects of ISO and other standards
#dimensions #geography #capturing
- Standardize geography and time dimensions for better data integration
- Consider capturing uncertainty with temporal-geolocation information
#methods #methodologies #computation
- Methods should be documented for each observation
- Document computation methodologies
#agreements #sharing #governance
- Metadata governance, security, compliance requirements, search, and collaboration tags should be defined
- Policy changes may be needed for data sharing within governance/consent agreements
- Achieve interoperability while maintaining data sovereignty
- Contractual agreements may be necessary for data sharing
#claims #automated #certification
- Facilitate automated reporting of practices
- Implement an automated certification framework to validate claims without revealing private data
This post-event report was contributed by Ankita Raturi, assistant professor of agriculture and biological engineering at the Agricultural Informatics Lab & Open Ag Tech and Systems Center Purdue University; Rob Trice, founder of The Mixing Bowl and Better Food Ventures; and Drew Zabrocki, co-founder of Centricity by Semios, advisor, OATS Center at Purdue University and International Fresh Produce Association.

Views expressed do not necessarily reflect the opinions of all participating organizations.